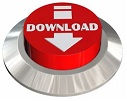
This paper focuses on developing an application for the mobile platform for peat fire reporting and a web-based application to collect peat fire location for decision-makers. This work’s results are the proposed application easy-to-use to monitoring potential peat fire by location and data activity. The exchangeable image (EXIF) file image and GPS metadata captured by a mobile phone can store and supply raw observation to our devices and sent it to the data center through global internet communication. In this paper, the global navigation satellite system (GNSS) and a global position system (GPS) sensor from a smartphone’s camera, is a useful tool to show the potential fire and smoke’s close-range location.
#Check photo geotag android#
Therefore, in this study, we develop an Android mobile platform application and a web-based application to support the citizen-volunteers who want to contribute wildfires reports, and the decision-makers who wish to collect, visualize, and evaluate these wildfires reports. The lack of peat fire detection systems is one factor that causing these reoccurring fires. Peat fires cause major environmental problems in Central Kalimantan Province, Indonesia and threaten human health and effect the social-economic sector. Our experiments show that the LPMP model can achieve comparable or better localization performance than NetVLAD and CoHog. Three main contributions are presented in this article: 1) the LPMP model is studied and compared with NetVLAD and CoHog, two state-of-the-art VPR models 2) a test benchmark for the evaluation of VPR models according to the type of environment traveled is proposed based on the Oxford car dataset and 3) the impact of the use of a novel detector leading to an uneven paving of an environment is evaluated in terms of the localization performance and compared to a regular paving.
#Check photo geotag code#
These two pieces of information are then merged to build a visuospatial code that is characteristic of the place where the visual scene was perceived.
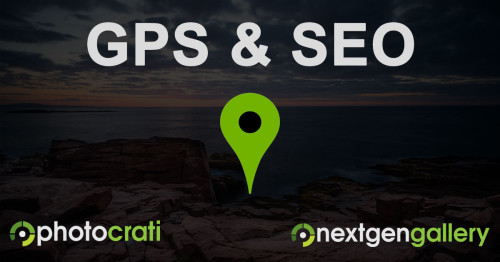
Inspired by the spatial cognition of mammals, visual information in the LPMP model are processed through two distinct pathways: a “what” pathway that extracts and learns the local visual signatures (landmarks) of a visual scene and a “where” pathway that computes their azimuth. This bio-inspired neural network allows building a neural representation of the environment via an unsupervised one-shot learning. To this end, the Log-Polar Max-Pi (LPMP) model is introduced. In this paper, we propose to tackle the VPR problem following a neuro-cybernetic approach.

The field of visual place recognition (VPR) aims to solve this challenge by relying on the visual modality to recognize a place despite changes in the appearance of the perceived visual scene. To illustrate, we present the performance gain in landmark retrieval and tag suggestion by utilising the accuracy-enhanced geo-metadata.Īutonomous vehicles require precise and reliable self-localization to cope with dynamic environments. Hence, for downstream applications, improved results can be obtained with these more accurate geo-metadata. Experimental results show that our proposed pyramid-based method outperforms its competitors and reduces orientation errors by an average of 58.8%.

To design a comprehensive model, we further incorporate smooth approximation and feature-based rotation estimation when formulating the error terms. As an alternative, we collect geographic context from OpenStreetMap and estimate the absolute viewing direction by comparing the image scene to world projections obtained with different external camera parameters. Given the small geo-distance between consecutive video frames, image-based localisation does not work due to the high ambiguity in the depth reconstruction of the scene. In this study, we focus on the challenging correction of compass readings and present an automatic approach to reduce these metadata errors. However, raw sensor data collected is often noisy, resulting in subsequent inaccurate geospatial analysis. Videos recorded with current mobile devices are increasingly geotagged at fine granularity and used in various location-based applications and services.
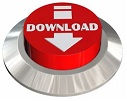